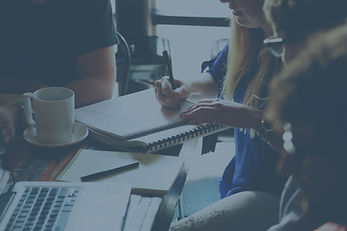
October 7
For solutions, purchase a LIVE CHAT plan or contact us
PSYCHOL 3020: Doing Research in Psychology: Advanced
Useful Background Readings
Kaplan, S. (1995). The restorative benefits of nature: Toward an integrative framework. Journal of environmental psychology, 15(3), 169-182.
doi:10.1016/0272-4944(95)90001-2
Djernis, D., Lerstrup, I., Poulsen, D., Stigsdotter, U., Dahlgaard, J., & O'Toole, M. (2019). A Systematic Review and Meta-Analysis of Nature-Based
Mindfulness: Effects of Moving Mindfulness Training into an Outdoor Natural Setting. Int J Environ Res Public Health, 16(17). doi:10.3390/
ijerph16173202
Holmes, E. A., & Mathews, A. (2010). Mental imagery in emotion and emotional disorders. Clin Psychol Rev, 30(3), 349-362. doi:10.1016/
j.cpr.2010.01.001
Marks, D. F. (1973). Visual imagery differences in the recall of pictures. British Journal of Psychology, 64(2), 17–24.
Menzel C, Reese G. Seeing nature from low to high levels: Mechanisms underlying the restorative effects of viewing nature images. PsyArXiv; 2021.
DOI: 10.31234/osf.io/e32vb.
Richardson, M., Passmore, H.-A., Lumber, R., Thomas, R., & Hunt, A. (2021). Moments, not minutes: The nature-wellbeing relationship.
International Journal of Wellbeing, 11(1).
Schultz, P. W. (2002). Inclusion with Nature: The Psychology Of Human-Nature Relations. In Psychology of sustainable development (pp. 61-78).
Boston, MA: Springer US.
Ulrich, R. S., Simons, R. F., Losito, B. D., Fiorito, E., Miles, M. A., & Zelson, M. (1991). Stress recovery during exposure to natural and urban
environments. Journal of environmental psychology, 11(3), 201-230. doi:10.1016/S0272-4944(05)80184-7
Part II Guide
Project Background
Sue is completing her honours thesis. She is particularly interested in the benefits of being in nature. Spending time
outdoors and feeling connected to the natural world is associated with measures of well-being (e.g. Capaldi et al.,
2014), mental health (e.g. Lackey et al., 2019), physical health (e.g. Stanhope et al., 2020), child development (e.g.
Guggenheim et al., 2012), pro-social behaviour (e.g. Putra et al., 2020) and pro-environmental behaviour (e.g. Martin et
al., 2020; Whitburn et al., 2020). Even indirect exposure to nature (e.g. videos and images) provides substantial benefits
for people who have restricted access to the natural environment (e.g. Djernis et al., 2019; Menzel & Reese, 2021). In a
seminal study on such indirect effects of Roger Ulrich (1991) showed that people confined to hospital beds healed faster,
required less pain medication, and were discharged sooner - if they had a window with a view of nature.
Specific autobiographical memory recall is
thought to be an ‘emotional amplifier’ linking
imagery to feelings experienced during similar
events in the past (Holmes & Mathews, 2010).
Thus, for her honours project, Sue is investigating
whether simply recalling a moment in nature can
elicit similar restorative effects without any
physical or visual nature-based stimulation. One
of the research questions Sue is addressing is:
Does reminiscing about moments in nature
increase feelings of restoration?
To probe if people feel more restored when
remembering a moment in nature, Sue has
designed a mental imagery task that involves
guiding participants through a series of questions
designed to help them immerse themselves in a
moment they bring to mind. In this experiment
Sue manipulates two things: the context or ‘place’
in which participants draw their moments and the
type of mental imagery intervention they use.
Participants are randomly assigned to one of two
“place” conditions: the first involves bringing a
moment to mind that is situated in a natural
environment (e.g., beach or mountains, a tree filled
park, or a backyard garden) and the second
involves bringing a moment to mind that is situated
in an urban environment (e.g., house or apartment
building, a city street, or a shopping mall). The
urban condition serves as the ‘control’ to the
nature condition. Participants are also randomly
assigned to one of two “intervention” conditions:
the first involves remembering a moment from the
past and the second involves imagining a new
moment. If nature-based mental imagery does
have restorative benefits, this second manipulation will
allow Sue to test if these benefits are dependent on
memory-based processes.
Your Task (Research Scenario)
Sue has designed a quantitative experiment to test the following research question: Does reminiscing about
moments in nature increase feelings of restoration? For Research Project Part II, consider what sort of aims or
research questions you might have that would explore this topic (broadly nature and wellbeing) from a
qualitative perspective. You can choose to develop broad statements of aims or very specific research questions;
we’ll look at the difference in class.
Participants in Sue’s experiment remembered or imagined a
moment in a natural or urban environment and then rated how
restored they felt on a scale from 0 (Not at all) to 100 (Entirely).
Directions:
You are to design a qualitative research design as per above. You should use the following headings and some
indicative word counts* are provided
INTRODUCTION: Provide a (very brief) introduction/ background to the problem you wish to address ending in aims
and/or research question/s. [This section should have at least four references] (approx. 300 words)
ETHICAL ISSUES: Provide a description of the ethical issues contained in your research (approx. 200 words)
METHODOLOGICAL APPROACH: (approx. 700 words all up). Provide an overview of the methods you would use
including:
• The overarching methodology: In this section you should include details on epistemology, overall type
of methodology (e.g., interpretative qualitative methodology; participatory action research; etc.). This
section should have at least two references for methodological details.
• Sample (if relevant – if you choose a discursive methodology for example you may not have a
participant sample): Sample details should include size, the population from which you wish to recruit,
and how you will conduct your recruitment.
• The data you will collect and how you will collect it: This section should include details on methods –
E.g. Focus groups, interviews, visual methods, etc. You should discuss why these methods are
suitable for your research question.
• How you will analyse your data: This section should include basic details about data analysis – e.g.
thematic analysis, discourse analysis, etc. You do not need to go into large amounts of detail but you
should discuss briefly what you will do – e.g. the steps you would take to analyse your data.
*word counts for individual sections are a GUIDE only. You could write more or less in any given section and still do
well provided you meet the criteria in the rubric.
============================================================================
FINC3017 Investments and Portfolio Management
Wednesday 26th October, 2022 11:59 pm.
Systematic risk (measured through MKT = rM,t − rf,t)
Size (measured through SMB)
Value (measured through HML)
Quality (measured through RMW)
Investment style (measured through CMA)
Momentum (measured through MOM)
Note that the data for all these factors is freely available from the Ken French data library. Exposures
to these factors will be measured through their respective betas. To study your portfolios, you have been
provided monthly returns from January 2016 until December 2020 for 100 stocks selected from the S&P 500
as well as their market capitalisations. This data will be used to estimate betas and associated prices of risk.
You also have daily return data for these 100 stocks from January 2021 until December 2021 (computed
from close-to-close). This data will be used to ascertain portfolio performance. Using this data, you are to
complete the following computational tasks:
1. Using monthly data from January 2016 (201601) to December 2020 (202012), compute the price of
risk (λj ) associated with each factor via a Fama-MacBeth regression. The time series estimation is
specified as
ri,t − rf,t = αi + βi,MKTMKT + βi,SMBSMBt + βi,HMLHMLt
+ βi,RMW RMWt + βi,CMACMAt + βi,MOMMOMt + i,t
Report your time series regression estimates and prices of risk in the highlighted areas of the Excel
solution template.
2. Using your results from the above regression, compute the weights for a long only risk parity portfolio
where weights are constructed from one of the factor betas via the expression
xi,F =
βi,F I(βi,F ≥ 0)
Pn
i=1 βi,F I(βi,F ≥ 0)
1
where xi,F is the weight allocated asset i where factor F = {MKT, SMB, HML, RMW, CMA, MOM}
and I is the indicator function:
I(C) =
1 if C is true
0 if C is false
This portfolio will be used to tilt your portfolio towards a specific factor to earn its associated risk
premium. You should pay special attention to the price of risk that each factor carries when deciding
which to use as your tilt. Report your market cap weights, factor portfolio weights in the highlighted
areas of your solution template.
3. Compute the initial weights for your overlaid portfolio. Recall that a factor overlaid portfolios weights
are given by
xF T = θxMC + (1 − θ)xF
where xF T is the allocation vector of your factor tilted portfolio, xMC is the market capitalization
weighted allocation vector and xF is the allocation vector for a risk parity portfolio constructed based
on one of the provided factors. The parameter θ represents how strongly you elect to tilt towards the
factor. Students are free to make this choice but must justify it. Report you chosen value of θ and the
overlaid portfolio weights in your solution template.
4. Using daily data from the start of January 2021 (20210104) until the end of December 2021 (20211231),
compute and plot the value of a $1 investment in your portfolio assuming that you can rebalance back
to your desired allocation (the original factor tilted weights) on a quarterly basis. This means that
you set your initial weights at the close on 20201231 (really the open of 20210104, but our dates aren’t
granular enough for that). You can then only alter the weights back to your original allocation on the
close of:
31st March, 2021 (20210331)
30th June, 2021 (20210630)
30th September, 2021 (20210930)
Also compute the value of a $1 investment in the market portfolio (you may use the Ken French market
as your proxy). Report your weights each day and the dollar value of your portfolio in the highlighted
areas of your solution template.
5. Evaluate the performance of your portfolio via the:
Arithmetic and geometric mean returns.
Holding period return.
Total risk (standard deviation of returns).
Downside risk.
alpha and beta.
Sharpe ratio.
Treynor ratio.
Sortino ratio.
95% historical VaR on a $1 notional.
==========================================================================
Scenario
The dataset is a modified version of one taken from the UCI Machine Learning Repository. The data
represent bank customers (and potential customers). For financial institutions, defaults (i.e., customers
who don’t pay back a loan) are a very important issue; being able to predict customers who will default
can be very important for operational and marketing purposes (e.g., whom we target, and to whom we
will give loans). Here, then, we are interested in understanding factors related to defaults, and
predicting which customers might default (the target variable). The attributes of each:
age (numeric) career: type of career (categorical) marital
: marital status (categorical) education (categorical)
balance: average yearly balance, in dollars (numeric)
num_loans: how many loans do they have with us? (numeric)
contact: contact communication type (categorical) day: last
contact day of the month (numeric) month: last contact
month of year (categorical) duration: last contact duration, in
seconds (numeric) contacts: number of times in contact with
the customer pdays: number of days that passed by after the
client was last contacted from a previous campaign
(numeric, -1 means client was not previously contacted) savings: does the
customer have a savings account with us? (binary: 1 or 0) default: has
defaulted? (binary: 1 or 0)
Question 1
Using techniques covered in class, which of the variables look like good candidates to help separate
defaulters from non-defaulters? For the two you consider good choices, include brief output supporting
this (e.g., one chart per variable), with one brief explanation for why these variables may be good
choices. Question 2
This dataset is very unbalanced. (Look at the distribution for ‘default’ to see this.) To see what happens
when we use an unbalanced dataset to build a model, we will first build a decision tree from the original
data. Use between 70% and 80% of the data (your choice) for your training set, 10% for the validation
set, and the remainder for the test set.
Include just your tree and your confusion matrices in your report.
Very briefly: How well does this model do? (You don’t need to perform any calculations—you should be
able to see very easily what is happening.) Why does this happen? (Looking at your tree may help you
understand this.)
Question 3
Now, build a decision tree from the same dataset, but balancing the data using weighting, according to
the rules that we have discussed. Show how you determined what size weights you used to balance
your set.
Use between 70% and 80% of the data (your choice) for your training set, 10% for the validation set, and
the remainder for the test set.
Include in your answer the tree diagram, the ‘split history’ diagram showing how the optimum size was
obtained, and the confusion matrices (training, test, and validation). For only the test set confusion
matrix:
• If you are using JMP Pro 14, you will need to correct for the weighting process, to determine the
performance for the original, unbalanced distribution. Show your calculations. (If you need
help with this, it is covered in the exercises document for the textbook.)
o If you are using JMP Pro 16, it seems to automatically correct for the weighting process.
You should double-check, however! If there are similar numbers of 1 and 0 instances in
the confusion matrix, then you are seeing ‘weighted’ results, and you will need to
‘reverse’ the weighting. If you are seeing many more 0 instances than 1s, then the
results have already been corrected.
• After doing so, calculate the correct classification rates, and interpret them (including both
precision and recall).
Interpret your results. Is this classifier doing a good job? Be careful to think of the nature of the data
and the business problem.
Question 4
Consider your decision tree. Can you make general statements about who is likely to default, based on
the rules in your tree? (Don’t simply restate every rule! Consider whether there are general insights to
be gained.)
Question 5
In the data file provided, there are 25 rows at the end with no label. Consider this data to be from new
or potential customers, for which we want to make predictions. (That’s one of the key purposes of our
work, after all!)
Of course, with a decision tree, we could manually make a prediction for each new customer, but that is:
a) slow; b) a lot of work!; c) error-prone. Moreover, for many of the models we will see later in the
course, it is much more cumbersome to make predictions manually. We would prefer to use the tool
itself.
One way to do so in JMP Pro is to manually type in new data rows at the end of the table; a much less
laborious process is the include the ‘new’ records at the end of the original file. (Of course, there are
means to ‘package’ the model and use it on completely new files, but we don’t need to do so here.)
New instances should have values for every field, except an empty ‘default’ field.
We can add columns that apply the model (in this case, the final decision tree) to every row in the file,
including the new ones. To do so, in the window displaying your decision tree output, click on the red
triangle menu (the one at the very top), and select Save Columns > Save Prediction Formula. After doing
so, you will see three new columns in your table: one each showing the predicted probability of
default=0 and default=1, and then a predicted value of ‘default’ itself. For labelled customers,
comparing these predictions to the real values of default is how we measure the accuracy of the model;
for new customers (which don’t have values for ‘default’!), we simply have the predictions. In your
report, include the following:
a) The last 25 rows in the table, showing the predictions for the ‘new’ instances;
b) Which customers are predicted to default?
c) What is the highest probability of default, and which customer(s) had it? Explain how that
probability is calculated. (Think about how we make decisions using a decision tree, and what
we get at the ‘end’ of a decision. Manually making a prediction using the tree may help, too.)
For solutions, purchase a LIVE CHAT plan or contact us
Follow us on Instagram and tag 10 friends for a $50 voucher! No minimum purchase required.